By GUPPI
What was the last time you actually set a foot in a bank? How often do you still pay with cash? I honestly cannot remember the last time I did any of those. Nowadays, online banking, card payments, online payments or paying by phone using apps such as PayPal are gaining traction (cfr. figure 1 & 2). Like any other sector, the financial sector was impacted by the drive towards more digitization.

Figure 1: evolution of the number of transactions across payment methods ranging from 2000 up to 2020^1

Figure 2: evolution of the transaction value in the digital payments sector ranging from 2017 up to 2025 (2022-2025 being a forecast)^2
The financial technology sector is becoming increasingly popular. Comparing the amount invested in fintech and the number of deals in H1’2021 to 2020, we see a notable growth. According to KPMG’s Pulse of Fintech report^3 (a bi-annual report on fintech investment trends), global venture capital investment in financial technology hit a record $52.3 billion in H1’2021, doubling the $22.5 billion reached in H2’2020. Now looking at the investments in AI and ML in the fintech industry specifically, we also can notice a soaring trend. The global AI in the fintech market was estimated at $7.91 billion in 2020, and it is expected to reach $26.67 billion by 2026, expecting a CAGR (Compound Annual Growth Rate) of 23.17% over the 5 year forecast period^4. However, this increase in popularity was ongoing already long before the COVID-19 crisis.
From where did this rise in popularity come? To give an answer to that, we should first travel back in time to the year 2008 when the worst economic catastrophe since the Great Depression of 1929 happened, namely the global financial crisis.
Let’s give a quick recap of this disaster. The financial crisis began with cheap credit, low interest rates and lax lending standards which fueled a housing bubble. Amidst this optimism, banks gave out loans to people with poor credit history (the so-called NINJA loans - no income, no job, no assets). These subprime mortgage-backed loans were then eventually repackaged and resold to investors as mortgage-backed securities and collateralized debt obligations. These turned out to be hugely profitable and so as to be able to meet ever-increasing demand, banks gave out loans to subprime borrowers. Finally, as borrowers started defaulting on their loans, more and more houses were put back on the market and housing supply met and surpassed demand leading to a collapse in housing prices. The bubble bursted. Mortgage-holders could not sell their houses for enough to cover their outstanding loans leading to high default rates and as a consequence, banks did not get their money back. This all eventually led to the fall of Lehman Brothers which marked the largest bankruptcy in U.S. history.
But why did this have such an impact on the emergence of fintech?
First, the global financial crisis led to large numbers of job losses in the financial sector and particularly amongst younger people. These then frequently pursued a new career by starting up a fintech company. Secondly, as a reaction to the financial crisis, governments imposed large numbers of regulatory changes designed to prohibit or alleviate the problems that caused this crisis. These regulations resulted in a dramatic increase in compliance and regulatory costs that only could be reduced via the use of new technology. The third impact the crisis had was a drop in trust in traditional financial institutions. People, especially the millennials, felt more comfortable doing financial transactions with a tech company rather than with a traditional bank. This opened many doors for fintech startups and eventually led to its popularity.
As previously mentioned, AI and ML techniques in fintech are booming too. Let’s now have a look at some of the AI applications in the financial industry.
Robo-advising and portfolio optimization
The first one we will briefly discuss is robo-advising and portfolio optimization. A robo advisor is a digital platform that provides automated and algorithmic-driven financial advice to customers usually following modern portfolio theory. Most robo-advisors will invest assets in mutual funds or ETFs (exchange traded fund) which spreads out risk (because of diversification) at a low cost, however, asset allocation will be customized and determined based on your investment goals. Typically, a customer starts by filling in a short survey regarding their current financial situation and their future aspirations. This data will then be used by the robo advisor to offer advice and automatically invest their client’s assets. Furthermore, most robo advisors nowadays can also perform tax-loss harvesting which enables clients to reduce the amount of taxes they have to pay on the capital gains.
Algorithmic trading

Algorithmic trading is about automating the trading of stocks and allows for faster and easier execution of orders. Machine learning algorithms can analyze historical trading patterns and predict market movements increasing the profitability of these traders. Furthermore, sentiment analysis can be performed to detect market sentiment (and hence the direction of the stocks) by analyzing social media comments (such as tweets about a particular company) and news headlines. This information can be used to complement the historic trading patterns and hence will improve the reliability of the prediction in market movements.
Anti Money Laundering (AML) & Know Your Customer (KYC)
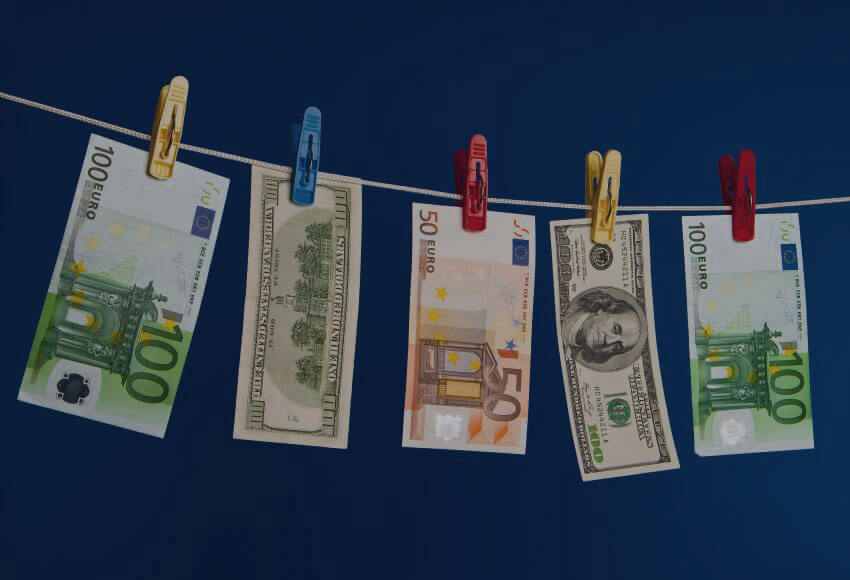
AML laws and regulations came into effect to prevent criminal activities such as market manipulation, trade in illegal goods, tax evasion, terrorist financing etc. from happening.
To help banks comply with AML regulations, anomaly detection algorithms can be run to detect and flag suspicious transaction behavior. The algorithm will analyze the historical transaction behavior of the clients and will flag suspicious behavior deviating from expected behavior learned from past transactions.
KYC on the other hand is part of AML and checks whether a customer is really who they say they are. This thus involves customer screening and identity verification.
To improve KYC solutions, AI can help perform document verification to authenticate the identity of an individual. It can check whether the document is authentic and if it is for instance not photoshopped or manipulated in any other way. Additionally, biometric verification can be improved with the use of AI by for instance running a 3D liveness detection algorithm to mitigate face spoofing attacks.
Fraud detection

While related to the AML regulations, we will discuss another type of fraud that is currently on the rise, namely payment card fraud. As the COVID-19 crisis pushes customers to increasingly use their cards rather than cash to reduce the transmission of the virus, payment card fraud unfortunately is also prospering. As payment card fraud is very expensive (in 2019, the global payment card fraud losses amounted to $28.65 billion^5 and as the number of transactions that occur simultaneously are rocketing, there is a need for automated fraud detection.
A typical AI model will analyze a customer’s spending habit using historical data and will flag transactions when these seem off and deviate from his normal behavior. Leveraging network information (between for instance the customers and the merchants) can also be interesting to consider in this case as fraudsters tend to be connected (which in social sciences is called the homophily effect).
Credit scoring
A credit score reflects the creditworthiness of a person, i.e. the ability to borrow money. This credit score is used to determine whether to extend or deny credit and influences the interest rate paid on the loan. Credit scoring is not only important for the traditional ways of lending, but also becomes increasingly important in peer-to-peer and marketplace lending.
AI can help improve the accuracy of these credit scores by considering employment, credit history, current income, work experience, transaction history and ability to earn more in the future.
InsurTech
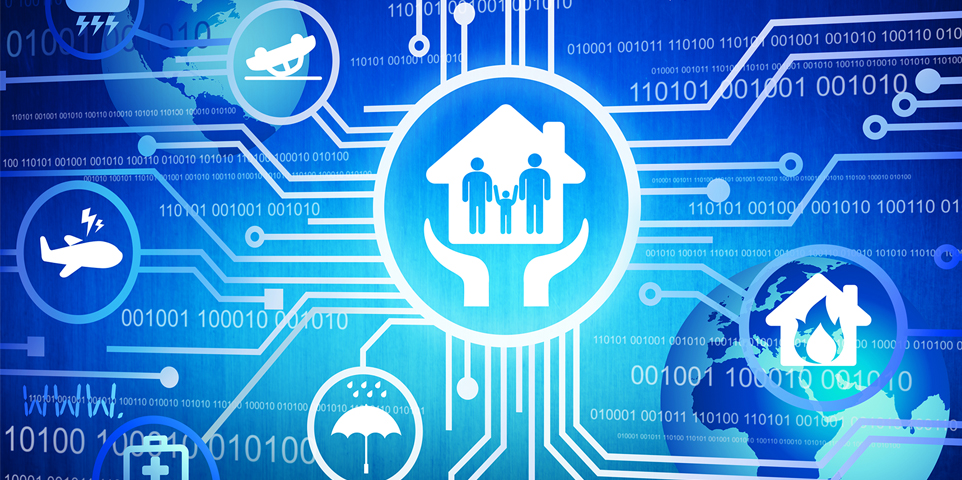
InsurTech refers to a subset of fintech that aims at increasing the efficiency in the insurance industry by the use of technology.
In insurance, predicting the risk a customer brings is crucial. AI can leverage a variety of data sources such as social media information, geospatial data, video and image data, IoT data etc. to better predict the riskiness of a client. Customer experience can also be enhanced by automatically triaging claims to the right people or detecting fraudulent claims using Natural Language Processing algorithms.
Computer vision can be used to speed up claims processing. For instance, it can assess damage due to floods, hurricanes or other natural disasters and provide an estimate of repair costs. Optical Character Recognition on the other hand can accelerate the onboarding process: a client simply uploads his ID card and the relevant information is automatically extracted from it so that a client profile can be easily built.
Additionally, AI can enhance the current claims fraud detection systems by flagging suspicious behavior by leveraging anomaly detection algorithms.
RegTech and compliance

RegTech is a subset of fintech whose purpose is to help businesses comply with regulations quickly and inexpensively. As previously mentioned, resulting from the global financial crisis of 2008, regulatory change has increased by approximately 500%. Many financial institutions are struggling to keep up with the pace of these regulations taking effect. This eventually leads to much higher operating costs for banks, sometimes even increasing these costs by 60%^6. NLP could help out here by extracting the relevant information from these regulatory documents and highlighting the entities on which these apply.
Outlook & conclusion
With the COVID-19 pandemic hitting hard, national lockdowns being announced, social distancing measures being imposed and many people being quarantined much of our daily lives moved online. This has fueled the trend towards digitization even more. According to McKinsey’s Global Survey of executives, the COVID-19 crisis accelerated the digitization of internal as well as external business processes by three to four years^7. This of course will drive further digitization of the financial sector.
While e-commerce has been booming the past years due to the obligated closure of shops, a rapid surge in online payments transpired. Additionally, payment card transactions have been up to reduce the spread of the virus and online banking became more popular. Hence, the growing use of digital financial services can turn a crisis into an opportunity to further grow.
Emerging from a financial crisis and accelerated by a health crisis, the use of technology in finance, including artificial intelligence and machine learning as a prominent innovation, remains a very interesting area to watch and grow in the coming years. The decision for financial institutions to adopt AI will be accelerated by technological advancement, increased user acceptance, and shifting regulatory frameworks. AI already has proven its value in use cases such as robo-advising, algorithmic trading, RegTech, InsurTech, fraud detection etc. Given that the field of AI is rapidly changing and that algorithms are improving every day and given the increased compute power available (f.i. cloud computing), the outlook of the use of AI in the fintech sector looks very bright.
References
- https://www.ecb.europa.eu/press/pr/stats/paysec/html/ecb.pis2020~5d0ea9dfa5.en.html
- https://www.statista.com/outlook/dmo/fintech/digital-payments/worldwide#transaction
- https://home.kpmg/xx/en/home/industries/financial-services/pulse-of-fintech.html
- https://www.mordorintelligence.com/industry-reports/ai-in-fintech-market
- https://nilsonreport.com/mention/1313/1link/
- https://databricks.com/blog/2021/09/16/4-ways-ai-can-future-proof-financial-services-risk-and-compliance.html
- https://www.mckinsey.com/business-functions/strategy-and-corporate-finance/our-insights/how-covid-19-has-pushed-companies-over-the-technology-tipping-point-and-transformed-business-forever
Images:

https://github.com/QuantessentiallyMe/social_security_5000 https://www.lehnerinvestments.com/en/algorithmic-trading-introduction-automated-trading/
https://paytechlaw.com/en/new-money-laundering-ordinance-3-0-amla/
https://siliconcanals.com/news/top-fraud-detection-startups-in-europe-in-2020e/
https://www.istockphoto.com/photos/credit-scoring
https://www.iii.org/article/background-on-insurtech
https://www.hedgethink.com/regtech-why-it-is-important-for-financial-services-firms-by-simon-pearson/